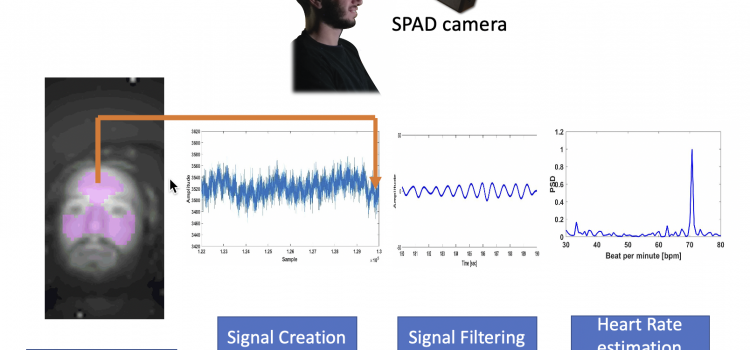
Yesterday, Marco Paracchini successfully defended his Ph.D. dissertation titled “Remote Biometric Signal Processing based on Deep Learning using SPAD Cameras.”
In his study, Marco aims at developing a compact system able to remotely and in real-time check the health condition of a person (heart rate, etc.) just by using a camera. This could be used in the automotive field in order to monitor the driver’s health state.
This thesis is part of the Horizon 2020 DEIS project!
Congratulations!
Abstract
Remote PhotoPlethysmoGraphy (rPPG) allows the extraction of cardiac information just by analyzing a video stream of a person face. In particular, the blood flowing in the vessels underneath the subject’s face introduces variations on the light intensity reflected by the skin that could be analyzed in order to obtain information about the subject’s heart activity. In this research, the adoption of a rPPG application in an automotive environment is investigated in order to monitor, in a non invasive fashion, the driver’s health state and potentially avoid accidents caused by acute illness states. The main goal of this work is to study and develop a rPPG system able to estimate numerous biomedical measurements in real time and in a dependable fashion. Moreover, this work explores the possibility of adopting a SPAD (i.e. Single-Photon Avalanche Diode) array camera instead of traditional RGB camera. In order to compensate for the SPAD camera’s low spatial resolution, a novel facial skin segmentation method, based on a deep learning model, is proposed. This method can precisely associate a skin label to each pixel of a given image depicting a face even when working with low resolution grayscale face images (64×32 pixel) and is able to work in presence of general environment condition regarding illumination, facial expressions, object occlusions and regardless of the gender, age and ethnicity of the subject. In order to perform and validate biometric measurements with a SPAD camera and compare it to estimations that could be obtained from a traditional RGB camera, multiple experiments have been conducted using a portable ECG device for reference. Moreover, some metrics were developed in order to monitor the dependability of the heart rate estimation and detect situations where an optical solution, such as rPPG, could fail. Finally, a rPPG application has been developed able to run in real time on a small ARM device equipped on a car. After receiving data from the SPAD camera, it is able to execute in real time the deep learning based pulse signal extraction and analyze it in order to constantly monitor the driver’s health condition by estimating multiple biometric parameters.